Laplace's law of succession
As of the 23rd May 2022 this website is archived and will receive no further updates.
understandinguncertainty.org was produced by the Winton programme for the public understanding of risk based in the Statistical Laboratory in the University of Cambridge. The aim was to help improve the way that uncertainty and risk are discussed in society, and show how probability and statistics can be both useful and entertaining.
Many of the animations were produced using Flash and will no longer work.
Suppose that every time there is an opportunity for an event to happen, then it occurs with unknown probability [math]p[/math]. Laplace's law of succession states that, if before we observed any events we thought all values of [math]p [/math] were equally likely, then after observing [math]r[/math] events out of [math]n[/math] opportunities a good estimate of [math]p[/math] is [math]\hat{p} = (r+1)/(n+2)[/math].
When the event keeps on occurring
Suppose we have observed [math]n [/math] events in [math]n [/math] opportunities. Then the probability of this sequence is [math]p^n[/math]. We need to turn this into a distribution for [math]p[/math] that represents reasonable belief: this requires Bayes theorem but, if we believe all value of [math]p [/math] were equally plausible before making any observations, the distribution for [math]p [/math] is just proportional to [math]p^n[/math]. Probability distributions must integrate up to 1, and [math]\int_0^1 p^n dp= 1/(n+1)[/math], and so the full probability distribution for [math]p [/math] must be of the form
[math]f(p) = (n+1)\,p^n[/math], drawn below for different values of [math] n[/math].
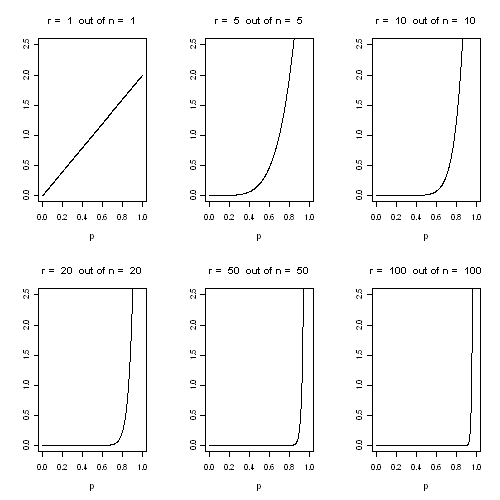
Probability distribution for various values of the chance [math]p [/math] of an event occurring, following [math]n [/math] events in [math]n [/math] opportunities
We can see that as the event occurs again and again, we become more confident that the underlying chance is near 1. To find the expectation of this distribution, we need to work out
[display]\int_0^1 \,\,p \,\,f(p) dp = (n+1) \,\,\int_0^1 \,\,p^{n+1} \,\,dp= \frac{(n+1)}{(n+2)}[/display].
When the event does not occur every time
Suppose we have observed [math]r [/math] events in [math]n [/math] opportunities. Then the probability of this particular sequence is [math]p^r \,\,(1-p)^{n-r}[/math]. Again, if we believe all value of [math]p [/math] were equally plausible before making any observations, the distribution for [math]p [/math] is just proportional to [math]p^r \,(1-p)^{n-r}[/math]. Probability distributions must integrate up to 1, and a standard result is that
[display]\int_0^1 \,\, p^r \,(1-p)^{n-r} dp = \frac{r!(n-r)!}{(n+1)!}. [/display]
So the full probability distribution for [math]p [/math] must be of the form
[display]f(p) = \frac{(n+1)!}{r!(n-r)!} \,\,p^r \,\,(1-p)^{n-r},[/display]
drawn below for different values of [math] r[/math] and [math] n[/math].
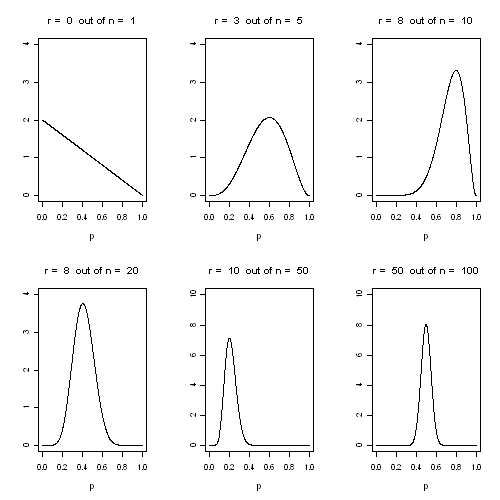
Probability distribution for various values of the chance [math]p [/math] of an event occurring, following [math]r [/math] events in [math]n [/math] opportunities (note change in vertical scale)
To find the expectation of this distribution, we need to work out
[display]\int_0^1 \,p \,f(p) dp = \frac{(n+1)!}{r!(n-r)!} \int_0^1\,\,p^{r+1} \,(1-p)^{n-r} dp= \frac{(n+1)!}{r!(n-r)!} \,\, \frac{(r+1)!(n-r)!}{(n+2)!}= \frac{(r+1)}{(n+2)}[/display].
- Log in to post comments
Comments
Anonymous (not verified)
Mon, 10/08/2009 - 10:55pm
Permalink
This post would have been